Share
Artificial intelligence (AI), also known as machine intelligence, refers to the capability of a machine to imitate human intelligence. It is now ubiquitous in everyday life, from the automated tagging of faces on Facebook to driverless cars. In the very near future, AI may also assist in the early diagnosis of glaucoma.
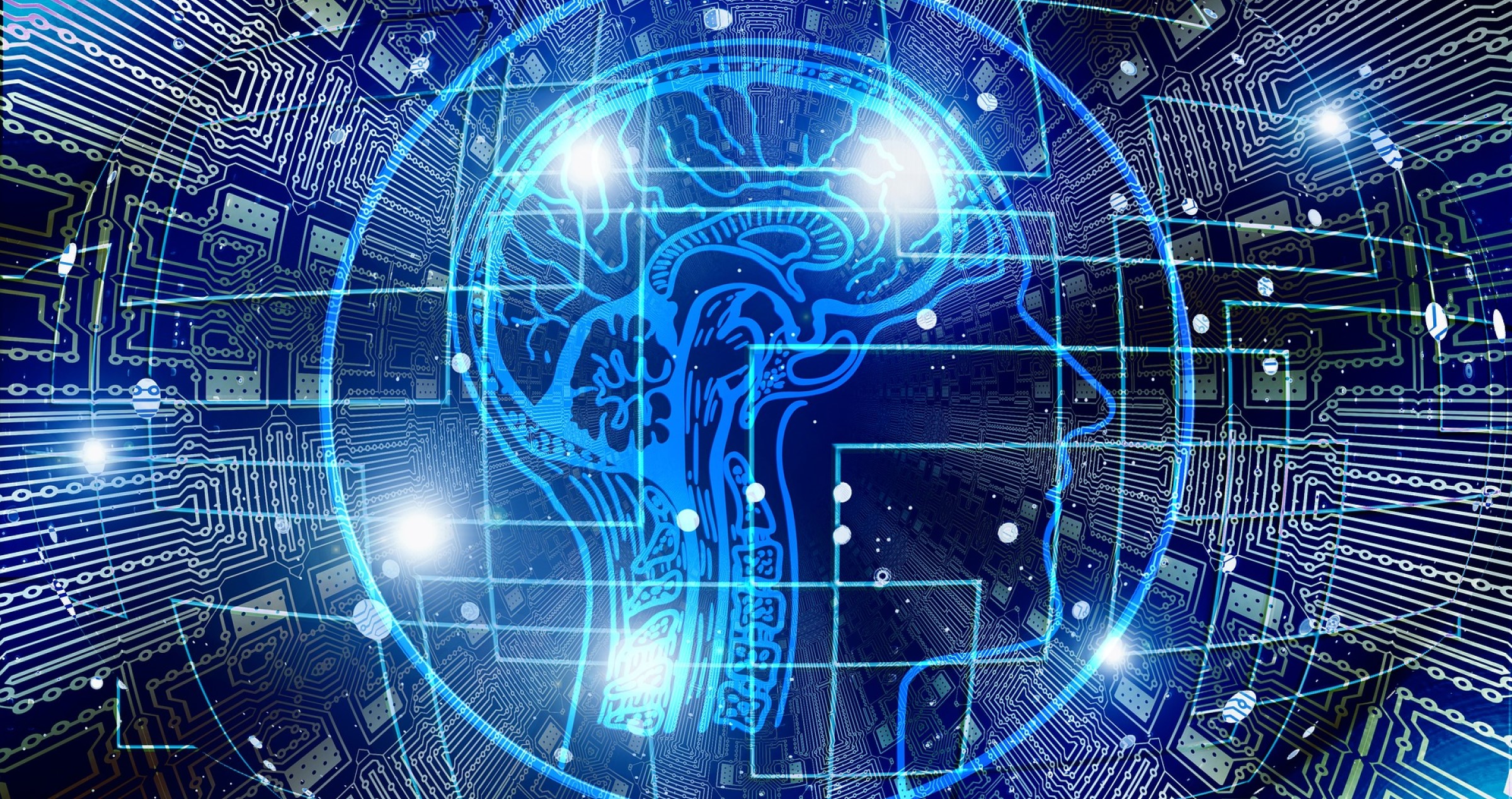
The rise of AI has been exponential over the past five years and follows advances in computer power, data volume, and theoretical understanding. AI techniques are now an essential part of the technology industry.
As electronic medical records become mainstream and digital image acquisition and data storage evolve, we will have more opportunities to access clinical data and patient images than ever before. An emerging area of diagnostic imaging in ophthalmology involves the use of automated computer vision image interpretation with deep learning algorithms. This involves training the algorithm on large datasets of well labelled images so that it can learn the features from the data itself rather than from predefined rules.
Sets of algorithms inspired by neural activity in the human brain, called ‘neural networks’, are designed to recognise patterns such as vertical lines in an image. Neural networks allow data to pass through layers of algorithms in a multistep process of pattern recognition to produce an output.1 Each step in deep learning allows the program to continually learn and evaluate its progress to reach a specific outcome.2 This technique lends itself particularly to fields that are highly image driven and therefore has not been limited to use in ophthalmology but has also been applied to dermatology and radiology.
AI And Glaucoma
Glaucoma is the leading cause of irreversible blindness worldwide.3,4,5 It is currently estimated that 64.3 million individuals are affected by this disease in the world,5 and this number is anticipated to reach 112 million by 2040.5 Blindness in glaucoma can be avoidable if the disease is detected early enough and treatment strategies commenced.6-8 However, ≥50 per cent of cases with glaucoma are undiagnosed in most population surveys around the world.9-14 This is attributable to glaucoma being largely asymptomatic until late in the disease process.
Glaucoma diagnosis is dependent on assessment of the optic disc and the surrounding retinal nerve fibre layer by a trained specialist. Therefore glaucoma screening has always been limited in its cost-effectiveness due to the skills required and its labour intensive nature.15,16 Given the growing public health concern, improvements for screening and diagnosis of glaucoma are warranted. AI, through the use of automated computer vision image interpretation with deep learning algorithms, offers a novel method of diagnostic imaging.
Different research groups around the world have analysed machine learning models for the diagnosis of glaucoma using available data such as visual fields, optical coherence tomography (OCT), and optic disc characteristics, and demonstrated excellent sensitivity, specificity, and area under the receiver operative characteristic curve (AUC) (Table1).17-29 Researchers at the Centre for Eye Research Australia (CERA) have formed a collaboration with the technology company Healgoo Interactive Med-Tech (Guangzhou, China) to develop a deep learning system based on an image data pool of close to 50,000 images collected from a range of clinical settings in China. A gold standard grading of each of these images was assigned based on the consensus reached by three individual glaucoma specialists. The deep learning algorithm was validated against an independent sample of 8,000 images and achieved an overall diagnostic accuracy in excess of 90 per cent (sensitivity = 95.6 per cent, specificity = 92.0 per cent) for the classification of referable glaucomatous optic neuropathy from standard colour 45° fundus photographs.21
Although still in its relative infancy in the specialty of glaucoma, the United States Food and Drug Administration (FDA) has already approved the first AI system for use in screening for diabetic retinopathy.30 We believe that, before long, the same will be seen for glaucoma.
However, the complexity of glaucoma diagnosis poses some unique challenges for AI systems that differ from retinal disease. In particular, population differences in fundus pigmentation, disc size, and cup to disc ratio are a potential source of bias for these systems. Tilted and myopic discs can also be problematic. These challenges can potentially be overcome with a diverse training dataset. Furthermore, while current deep learning algorithms rely on training using single modality imaging such as fundus photography, and subjective grading by specialists to label images (that can have its own limitations), the future is likely to see improved accuracy through the addition of real world clinical data. Optical coherence tomography and automated visual fields, for example, will improve automated glaucoma classification. Ultimately, given glaucoma progression and treatment response can vary markedly across patients, we would like to see this leading on to improved risk prediction and personalised treatment for individual patients. It is anticipated that the use of longitudinal data may assist in classifying cases into predictive outcomes and determine likelihood of treatment response.
References
1. Artificial Intelligence, Machine Learning and Deep Learning. Deepleaning4J (DL4J). 2017.
2. Kapoor R, Walters SP, Al-Aswad LA. The current state of artificial intelligence in ophthalmology. Survey of ophthalmology. 2018.
3. Stevens GA, White RA, Flaxman SR, et al. Global prevalence of vision impairment and blindness: magnitude and temporal trends, 1990-2010. Ophthalmology. 2013;120(12):2377-2384.
4. Bourne RR, Stevens GA, White RA, et al. Causes of vision loss worldwide, 1990-2010: a systematic analysis. Lancet Glob Health. 2013;1(6):e339-349.
5. Tham YC, Li X, Wong TY, Quigley HA, Aung T, Cheng CY. Global prevalence of glaucoma and projections of glaucoma burden through 2040: a systematic review and meta-analysis. Ophthalmology. 2014;121(11):2081-2090.
6. Hood DC, Raza AS, de Moraes CG, Liebmann JM, Ritch R. Glaucomatous damage of the macula. Prog Retin Eye Res. 2013;32:1-21.
7. Tatham AJ, Medeiros FA, Zangwill LM, Weinreb RN. Strategies to improve early diagnosis in glaucoma. Prog Brain Res. 2015;221:103-133.
8. Tatham AJ, Weinreb RN, Medeiros FA. Strategies for improving early detection of glaucoma: the combined structure-function index. Clin Ophthalmol. 2014;8:611-621.
9. Baskaran M, Foo RC, Cheng CY, et al. The Prevalence and Types of Glaucoma in an Urban Chinese Population: The Singapore Chinese Eye Study. JAMA Ophthalmol. 2015;133(8):874-880.
10. Varma R, Ying-Lai M, Francis BA, et al. Prevalence of open-angle glaucoma and ocular hypertension in Latinos: the Los Angeles Latino Eye Study. Ophthalmology. 2004;111(8):1439-1448.
11. Quigley HA, West SK, Rodriguez J, Munoz B, Klein R, Snyder R. The prevalence of glaucoma in a population-based study of Hispanic subjects: Proyecto VER. Archives of ophthalmology. 2001;119(12):1819-1826.
12. Weih LM, Nanjan M, McCarty CA, Taylor HR. Prevalence and predictors of open-angle glaucoma: results from the visual impairment project. Ophthalmology. 2001;108(11):1966-1972.
13. Gupta P, Zhao D, Guallar E, Ko F, Boland MV, Friedman DS. Prevalence of Glaucoma in the United States: The 2005-2008 National Health and Nutrition Examination Survey. Investigative ophthalmology & visual science. 2016;57(6):2905-2913.
14. Keel S, Xie J, Foreman J, et al. Prevalence of glaucoma in the Australian National Eye Health Survey. The British journal of ophthalmology. 2018.
15. Moyer VA, Force USPST. Screening for glaucoma: U.S. Preventive Services Task Force Recommendation Statement. Ann Intern Med. 2013;159(7):484-489.
16. Fleming C, Whitlock EP, Beil T, Smit B, Harris RP. Screening for primary open-angle glaucoma in the primary care setting: an update for the US preventive services task force. Ann Fam Med. 2005;3(2):167-170.
17. Singh A, Dutta MK, ParthaSarathi M, Uher V, Burget R. Image processing based automatic diagnosis of glaucoma using wavelet features of segmented optic disc from fundus image. Comput Methods Programs Biomed. 2016;124:108-120.
18. Salam AA, Khalil T, Akram MU, Jameel A, Basit I. Automated detection of glaucoma using structural and non structural features. Springerplus. 2016;5(1):1519.
19. Chakrabarty L, Joshi GD, Chakravarty A, Raman GV, Krishnadas SR, Sivaswamy J. Automated Detection of Glaucoma From Topographic Features of the Optic Nerve Head in Color Fundus Photographs. J Glaucoma. 2016;25(7):590-597.
20. Xiangyu C, Yanwu X, Damon Wing Kee W, Tien Yin W, Jiang L. Glaucoma detection based on deep convolutional neural network. Conf Proc IEEE Eng Med Biol Soc. 2015;2015:715-718.
21. Li Z, He Y, Keel S, Meng W, Chang RT, He M. Efficacy of a Deep Learning System for Detecting Glaucomatous Optic Neuropathy Based on Color Fundus Photographs. Ophthalmology. 2018;125(8):1199-1206.
22. Asaoka R, Murata H, Iwase A, Araie M. Detecting Preperimetric Glaucoma with Standard Automated Perimetry Using a Deep Learning Classifier. Ophthalmology. 2016;123(9):1974-1980.
23. Muhammad H, Fuchs TJ, De Cuir N, et al. Hybrid Deep Learning on Single Wide-field Optical Coherence tomography Scans Accurately Classifies Glaucoma Suspects. J Glaucoma. 2017;26(12):1086-1094.
24. Oh E, Yoo TK, Hong S. Artificial Neural Network Approach for Differentiating Open-Angle Glaucoma From Glaucoma Suspect Without a Visual Field Test. Investigative ophthalmology & visual science. 2015;56(6):3957-3966.
25. Bowd C, Lee I, Goldbaum MH, et al. Predicting glaucomatous progression in glaucoma suspect eyes using relevance vector machine classifiers for combined structural and functional measurements. Investigative ophthalmology & visual science. 2012;53(4):2382-2389.
26. Niwas SI, Lin W, Bai X, et al. Automated anterior segment OCT image analysis for Angle Closure Glaucoma mechanisms classification. Comput Methods Programs Biomed. 2016;130:65-75.
27. Silva FR, Vidotti VG, Cremasco F, Dias M, Gomi ES, Costa VP. Sensitivity and specificity of machine learning classifiers for glaucoma diagnosis using Spectral Domain OCT and standard automated perimetry. Arq Bras Oftalmol. 2013;76(3):170-174.
28. Vidotti VG, Costa VP, Silva FR, et al. Sensitivity and specificity of machine learning classifiers and spectral domain OCT for the diagnosis of glaucoma. Eur J Ophthalmol. 2012:0.
29. Li Z, Keel S, Liu C, He M. Can Artificial Intelligence Make Screening Faster, More Accurate, and More Accessible? Asia Pac J Ophthalmol (Phila). 2018;7:440-445.
30. Abramoff MDL, P.T.; Birch, M.; Shah, N.; Folk, J.C. Pivotal Trial of an autonomous AI-based diagnostic system for detection of diabetic retinopathy in primary care offices. npj Digital Medicine. 2018;1(39).